Unlocking Innovation: Comprehensive Guide to Training Data for Generative AI
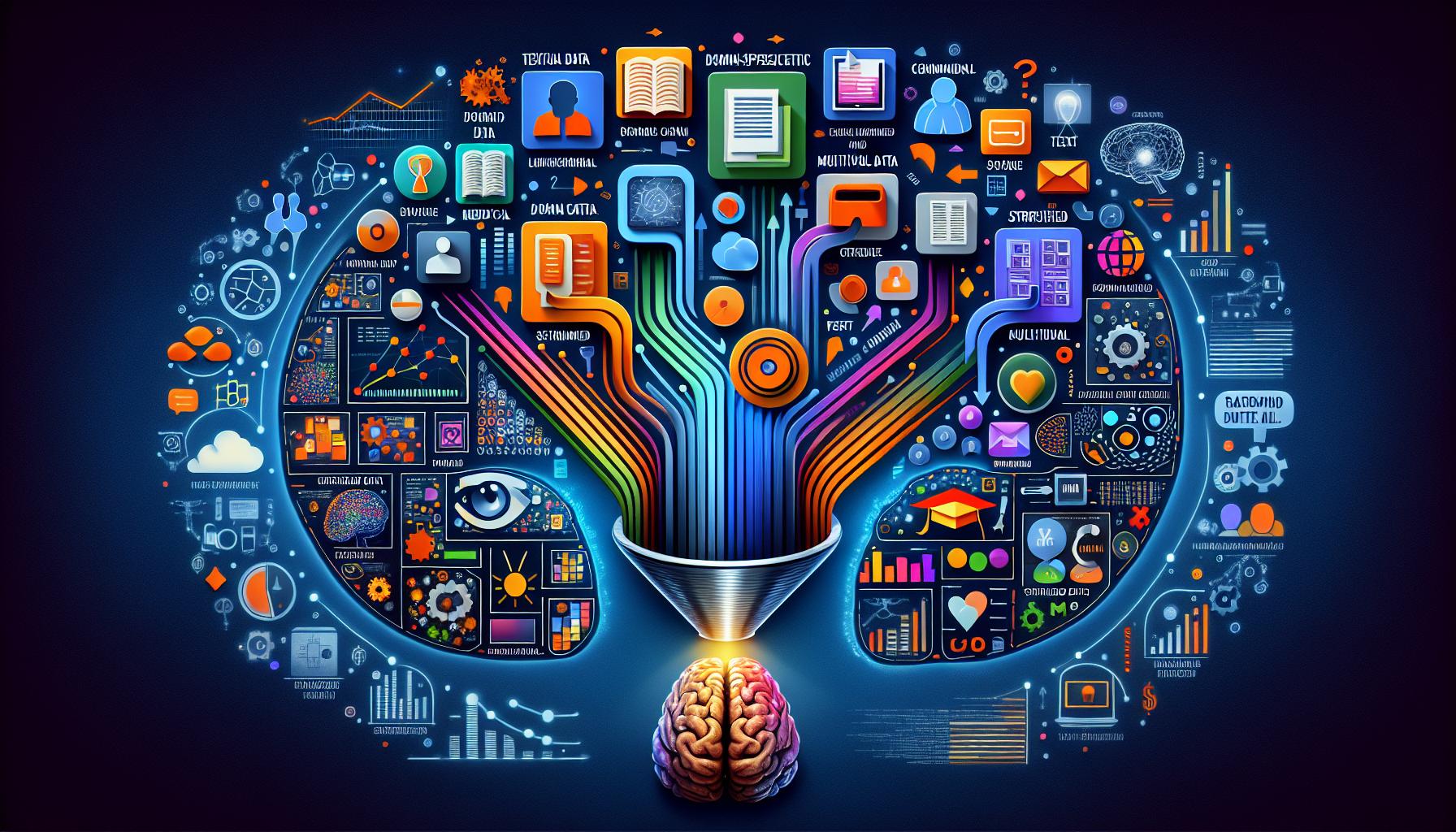
Introduction
High-quality training data is crucial for the success of generative A.I. models, which are capable of creating new and original content such as text, images, videos, and music. Understanding the intricacies of sourcing training data is essential for developing effective A.I. models. Generative A.I. models learn from extensive datasets to generate human-like content, and the quality, diversity, and quantity of this data significantly impact their performance.
Understanding Generative AI
Generative A.I. refers to a type of Artificial Intelligence that can generate new content by learning from previous examples. This technology automates complex tasks and enhances decision-making processes by providing insights beyond traditional data analysis methods. As the scope of training data evolves, it enables more personalized customer experiences and innovative content creation, transforming how companies interact with their audiences.
Role of Training Data
Training data is vital for generative A.I. models to understand patterns, grammar, context, and semantics, allowing them to produce coherent and contextually relevant content. The better the quality and diversity of the training data, the more accurate and versatile the A.I. model will be.
Types of Training Data
Text Data: Essential for text-generating models like GPT, sourced from books, articles, websites, and social media.
Domain-Specific Data: Used for specialized applications in fields like healthcare and finance to ensure contextually accurate outputs.
User-Generated Content: Includes social media posts and forum discussions, capturing informal language and diverse perspectives.
Multimodal Data: Combines text, images, audio, and video to enhance A.I. capabilities, useful for tasks like image captioning.
Structured Data: Structured formats like databases can be converted into textual content for reports and summaries.
Image Data: Vital for models like DALL-E that generate images from textual descriptions, sourced from public and private collections.
Best Practices for Sourcing Training Data
Diversify Sources: Use a wide range of data sources, including public datasets, proprietary data, and crowdsourced content.
User Consent and Bias Mitigation: Anonymize user data and address biases to ensure representative and unbiased training datasets.
Collaborations: Partner with businesses or researchers to access area-specific data, pooling resources for comprehensive datasets.
Data Preprocessing: Involve correcting errors, removing duplicates, and standardizing formats to ensure data quality.
Data Cleaning and Labeling: Invest in eliminating noise and ensuring accuracy in training data.
Data Generation: Use A.I. to create artificial data when real-world data is scarce, supplementing training datasets.
Continuous Learning: Regularly update training data to keep A.I. models current and robust, adapting to evolving language and emerging topics.
Outsourcing vs. Internal Sourcing
Companies face a choice between internal sourcing and outsourcing training data. Internal sourcing provides control but demands resources and expertise in data gathering and compliance with privacy policies. Outsourcing to specialized vendors like Macgence offers advantages like access to high-quality, diverse datasets while adhering to data privacy regulations. This approach allows companies to focus on model development and innovation.
Macgence's Role
Macgence offers comprehensive solutions for sourcing training data, including curated datasets and data annotation services, prioritizing ethical data sourcing. Partnering with Macgence helps businesses develop high-performing A.I. models while maintaining ethical standards and data privacy.
Conclusion
High-quality training data is imperative for developing effective generative A.I. systems, driving innovation, and maintaining a competitive edge. By employing best practices and considering outsourcing options, developers and business leaders can navigate the complexities of generative A.I. data sourcing to ensure their models are robust and data-smart.
Answers to Key Questions
What approaches can be used for bias mitigation in training data for generative AI?
To mitigate bias in training data for generative AI, several approaches can be adopted:
- Anonymization: Removing personally identifiable information to prevent models from learning bias associated with specific individuals.
- Bias Audits: Regularly checking datasets for imbalances and biases, correcting them as necessary.
- Diverse Data Sourcing: Ensuring data is collected from a variety of sources to reflect different demographics and perspectives.
- Feedback Mechanisms: Implementing systems where users can report bias, allowing for continuous improvement of datasets.
How does continuous learning contribute to the relevance and effectiveness of generative A.I. models?
Continuous learning plays a crucial role in maintaining the relevance and effectiveness of generative A.I. models by:
- Adapting to New Data: Regular updates incorporating new data ensure A.I. models stay current with evolving language and trends.
- Improving Accuracy: Ongoing learning helps models correct errors and refine outputs over time.
- Robustness: Models become more resilient to data anomalies and rare cases by continuously learning from diverse data points.
- Innovative Outputs: Continuous learning contributes to generating more creative and relevant content by leveraging the latest data insights.
What are the potential advantages of using multimodal data for training generative A.I. models?
Using multimodal data for training generative A.I. models offers several advantages:
- Enhanced Capabilities: Combining text, images, audio, and video enables A.I. models to perform complex tasks like image captioning and video summarization.
- Rich Context Understanding: Multimodal data provides comprehensive context, improving the relevance and coherence of generated content.
- Diverse Applications: Supports a wide range of applications, from virtual assistants to augmented reality experiences.
- Improved Personalization: Multimodal models can create more personalized content by considering various types of user input.