The Rise of AI Agent Infrastructure and the Crucial Role of RAIA as a Launch Pad
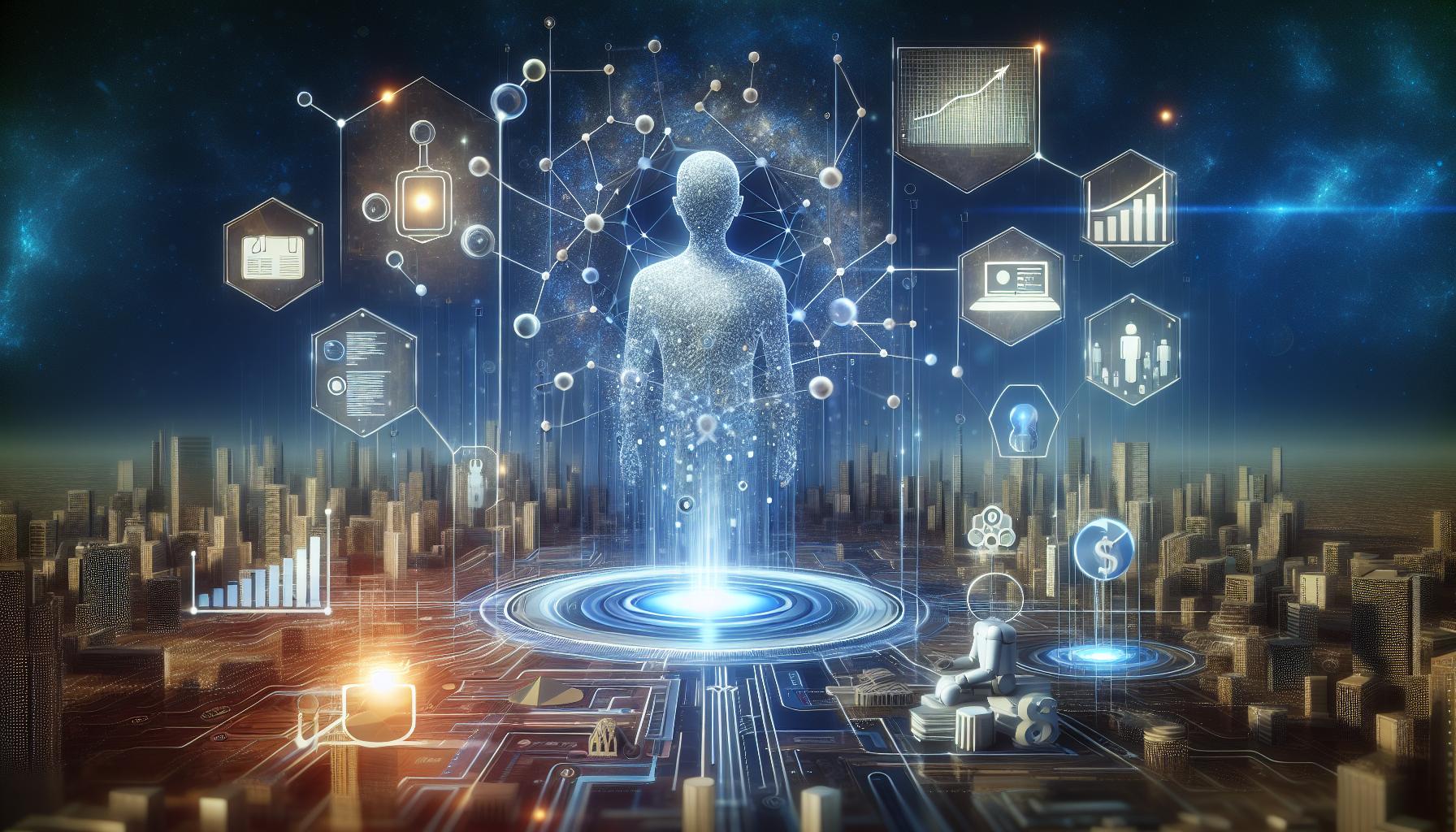
Introduction
In the past two years, the tech industry has witnessed a transformative boom in Generative A.I. (GenAI) applications. These applications span various domains such as productivity, development, cloud infrastructure management, media consumption, and even healthcare revenue cycle management. The driving force behind this surge is the rapidly evolving A.I. models supported by robust platform infrastructure. However, the accelerated pace of GenAI development presents new challenges. As developers build applications on a still-evolving foundation, the necessity for a streamlined, supportive infrastructure becomes apparent. This is where platforms like RAIA play a crucial role, helping businesses easily build, train, and test their A.I. applications.
The Rise of A.I. Agents
A significant shift in the A.I. landscape is the emergence of A.I. agents. These autonomous entities are capable of planning and executing multi-step tasks, simplifying interaction for end-users and providing a new abstraction for developers to build upon. Earlier frameworks like LangChain, LlamaIndex, and Semantic Kernel set the stage for these advancements, allowing A.I. agents to interact with APIs via code. Research from leading institutions has further indicated that A.I. agents perform better collectively than individually.
Types of A.I. agents include:
Hand-Crafted Agents:
These operate within narrow constraints, using chains of prompts and API calls.
Specialized Agents:
These have broader capabilities, dynamically deciding tasks within a subset of task types and tools.
General Agents:
Theoretically, these would perform any task but currently remain a future goal due to existing model limitations in reasoning capabilities.
Practical Applications of A.I. Agents
Despite their limitations, A.I. agents are becoming integral to various sectors. For instance:
Matrices:
A spreadsheet application uses agents to automate data entry and web searches based on inferred needs.
Gradial:
This marketing automation platform employs agents to manage content creation and updates, integrating seamlessly with systems like JIRA and Workfront.
Current Limitations and Future Potential
AI agents, while promising, are still imperfect. They often require supervision, management, and optimization to handle bandwidth, cost, latency, and user experience issues. Developers use various techniques like validation and model ensembles to enhance reliability and performance, anticipating rapid advancements in A.I. research.
Infrastructural Needs for Supporting A.I. Agents
The current state of A.I. agent infrastructure is characterized by a fragmented and vertically integrated landscape. Critical infrastructural elements include:
Agent-Specific Developer Tools:
Tools like Flowplay and Wordware help standardize design patterns, facilitating easier adoption and more robust agent development.
Agents as a Service:
Hand-crafted agents for specific tasks are becoming available as services, enabling developers to integrate them into broader applications via APIs.
Browser Infrastructure:
Browsers like Browserbase and Apify manage complex tasks like web scraping and API interactions, crucial for enriching agents with comprehensive data access.
Personalized Memory:
Vector stores like Pinecone and specialized memory functionalities like Cognee ensure agents have relevant historical context, enhancing their performance.
Authentication for Agents:
Platforms like Anon.com manage the security needs of agents, ensuring secure API interactions on behalf of end-users.
The Role of RAIA as a Launch Pad
In this evolving landscape, RAIA stands out as a critical launch pad for business users seeking to develop A.I. applications. RAIA simplifies the complex processes of building, training, and testing A.I. models. It offers a comprehensive infrastructure that can adapt to the rapidly changing A.I. environment. By providing managed services, RAIA allows developers to focus on innovation and product differentiation rather than infrastructural challenges.
Conclusion
The rise of A.I. agent infrastructure signifies a pivotal moment in application development. While the field is still maturing, the ongoing advancements promise a future where A.I. agents significantly enhance productivity across various industries. Platforms like RAIA are essential, offering the foundational support businesses need to navigate and thrive in this dynamic landscape.