The Impact of OpenAI and Large Language Models on the Future of AI Research
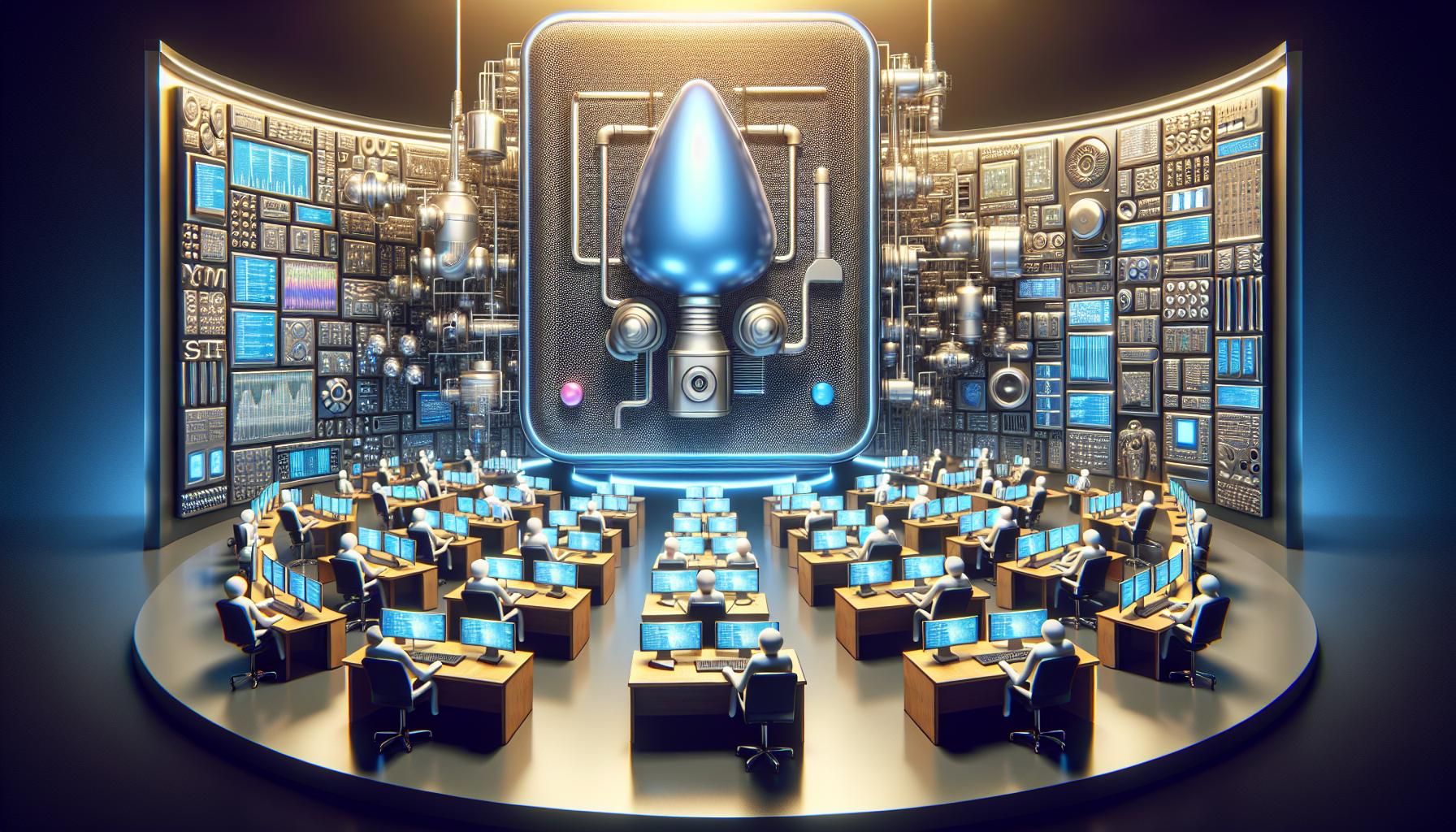
Introduction
The realm of Artificial Intelligence (AI) is witnessing rapid advancements, with large language models (LLMs) at the forefront of this revolution. However, some experts argue that this focus might be detrimental to the broader spectrum of A.I. research. In this blog, we will delve into a Google engineer's claims that OpenAI, under Sam Altman's leadership, has significantly hindered progress in A.I. research by placing undue emphasis on LLMs. We will explore the ways in which LLMs have overshadowed other areas of A.I. research, the potential setbacks for future developments, and alternative A.I. research pathways that may be neglected due to this focus.
The Rise of Large Language Models
Large language models like OpenAI's GPT series have dominated the A.I. landscape in recent years. These models, trained on vast amounts of text data, excel in generating human-like text and have found applications in various domains, from chatbots to content creation. The success of these models has led to significant investments and research efforts being channeled into further enhancing their capabilities.
Overshadowing Other Areas of A.I. Research
The Google engineer's assertion that LLMs have overshadowed other A.I. research areas is rooted in several observations:
Narrow Focus on LLMs Stifles Innovation
As resources and attention are disproportionately directed towards improving LLMs, other critical areas of A.I. research receive less support. These areas include computer vision, reinforcement learning, symbolic AI, and multimodal A.I. systems, which integrate multiple forms of data such as text, images, and audio.
Decline in Diversity of Research Directions
The current trend favors research that builds on the existing LLM frameworks rather than exploring novel approaches. This can lead to a homogenization of A.I. research, where innovative and potentially groundbreaking ideas are sidelined in favor of incremental improvements to LLMs.
Resource Allocation and Opportunity Cost
Research institutions and companies often have finite resources, including funding, computational power, and talent. The heavy investment in LLMs means fewer resources are available for exploring other A.I. technologies. This opportunity cost may hinder the discovery of alternative A.I. methodologies that could offer unique advantages over LLMs.
Potential Setbacks for Future A.I. Developments
The preoccupation with LLMs could have several long-term consequences for A.I. research and innovation:
Slower Progress in Understudied Areas
Areas of A.I. research that are currently underfunded or underexplored may progress more slowly, delaying potential breakthroughs that could enhance the capabilities and applications of AI.
Increased Risk of Monoculture in AI
Focusing heavily on LLMs may create a monoculture in A.I. research, where the diversity of ideas and approaches is reduced. This lack of diversity can make the A.I. field less resilient to challenges and less capable of adapting to new problems.
Neglected A.I. Research Pathways
Several promising areas of A.I. research may be neglected due to the current focus on LLMs:
Explainable A.I. (XAI)
Explainable A.I. aims to make A.I. systems more transparent and understandable to humans. With the surge in LLM research, efforts to develop interpretable A.I. models that provide clear explanations for their decisions might be sidelined.
AI for Social Good
AI research geared towards addressing societal challenges, such as climate change, healthcare, and education, may struggle to attract attention and funding compared to the more commercially viable LLM projects.
Neurosymbolic AI
This area combines neural networks with symbolic reasoning to create A.I. systems that can understand and manipulate symbols and concepts. The potential of neurosymbolic A.I. to enhance cognitive abilities is significant, but it may be overlooked in favor of LLM advancements.
Conclusion
The Google engineer's perspective highlights the broader implications of the current A.I. research landscape's focus on large language models. While LLMs have demonstrated remarkable capabilities and potential, it is crucial to maintain a balanced approach to A.I. research that includes fostering diversity and innovation in less-explored areas. By doing so, the A.I. community can ensure sustainable and comprehensive progress across the entire spectrum of A.I. technologies.
Call to Action
As members of the A.I. community, researchers, policymakers, and stakeholders must advocate for a more diversified A.I. research agenda. Allocating resources and attention to underfunded and emerging areas of A.I. research can unlock new opportunities and drive the field forward in a way that benefits society as a whole. By recognizing and addressing the potential drawbacks of an LLM-centric approach, we can pave the way for a more inclusive and innovative A.I. future.