Revolutionizing Weather Forecasting: AI and the Future of Meteorology
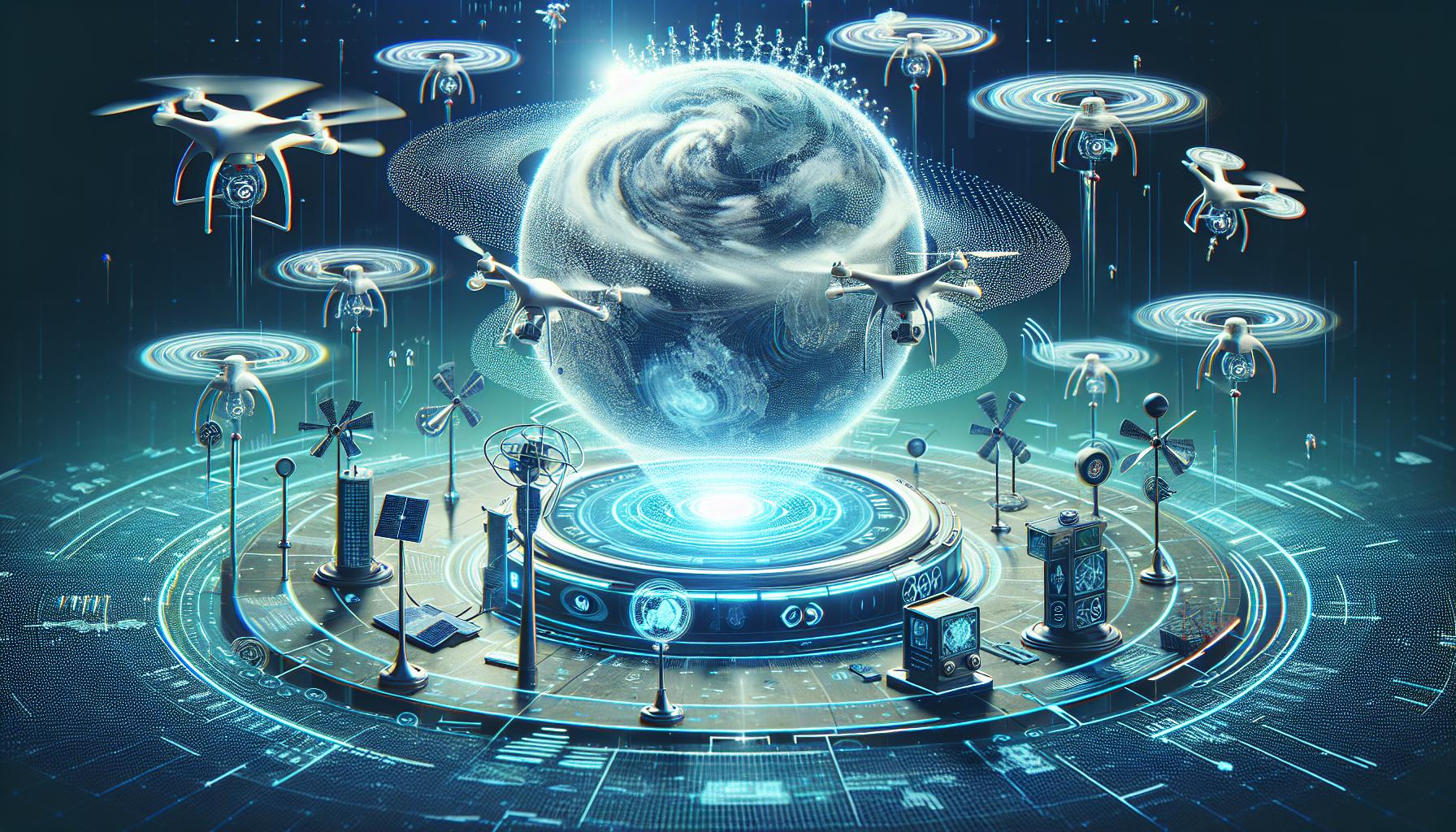
Revolutionizing Weather Forecasting: A.I. and the Future of Meteorology
Significant changes are underway in weather forecasting, driven by advances in Artificial Intelligence (AI). The goal is to revolutionize forecasting methods, making them accessible even on desktop computers. This transformation is anchored in the powerful capability of A.I. systems, such as large language models, that rely heavily on vast amounts of data for training.
The Data Revolution in Weather Prediction
One notable alternative data source is the ERA5 dataset provided by the European Centre for Medium-Range Weather Forecasts (ECMWF). ERA5 encompasses extensive atmospheric, land, and oceanic data, dating back to 1940, making it an invaluable resource for training A.I. models in weather prediction. The breadth and depth of the data enable A.I. models to learn complex weather patterns and improve forecasting accuracy. This shift in data utilization has yielded rapid advancements in AI-driven weather forecasting, with some models even outperforming traditional methods that require powerful supercomputers.
Pioneers in AI-Driven Weather Forecasting
WindBorne Systems, co-founded by Stanford graduates John Dean and Kai Marshland, exemplifies the transformative potential of A.I. in weather forecasting. They developed small, long-duration weather balloons designed to collect atmospheric data and address gaps in traditional weather data collection systems. Their A.I. weather model, WeatherMesh, has demonstrated superior performance in essential forecasting tasks, including hurricane prediction, outperforming traditional physics-based models. The innovation lies in their approach requiring significantly less computational power, underscoring AI's capability to revolutionize weather prediction systems.
The Role of ERA5 and Synthetic Data
ERA5, a robust repository of historical weather data, has been instrumental in training advanced A.I. models. Such datasets provide the rich, diverse information needed to hone the accuracy of forecasting models. Moreover, synthetic data generation techniques offer an additional layer of vast, realistic data that can augment existing datasets, enabling A.I. models to train on scenarios that may not be frequently recorded in real historical datasets. This combination of ERA5 and synthetic data significantly enhances an A.I. model's capacity to predict weather with greater precision and reliability.
Evident Advancements and Future Prospects
Early academic work and recent advancements emphasize the increasing efficiency of A.I. models in weather forecasting. Notably, the European weather center's AIFS model highlights the promising aspects of deep learning techniques applied to meteorology. These models continue to evolve, integrating data assimilation and forecasting processes, potentially leading to a fully revolutionary approach to weather prediction in the future. Furthermore, the continuous improvements in data quality and A.I. algorithms suggest that AI-driven weather models will become more accurate, reliable, and accessible, potentially democratizing weather prediction.
Conclusion
In conclusion, the intersection of A.I. and meteorology marks a significant leap towards advanced weather forecasting. With the integration of alternative data sources like ERA5 and synthetic data, A.I. models are set to outshine traditional supercomputer-dependent methods. The pioneering efforts of companies like WindBorne Systems showcase the practical applications and benefits of AI-driven weather models in real-world scenarios. The horizon looks promising as A.I. continues to mature, potentially offering a seamless blend of data assimilation and forecasting, paving the way for more accurate, dependable, and accessible weather predictions.