Iterative Excellence: The Continuous Improvement of AI Models
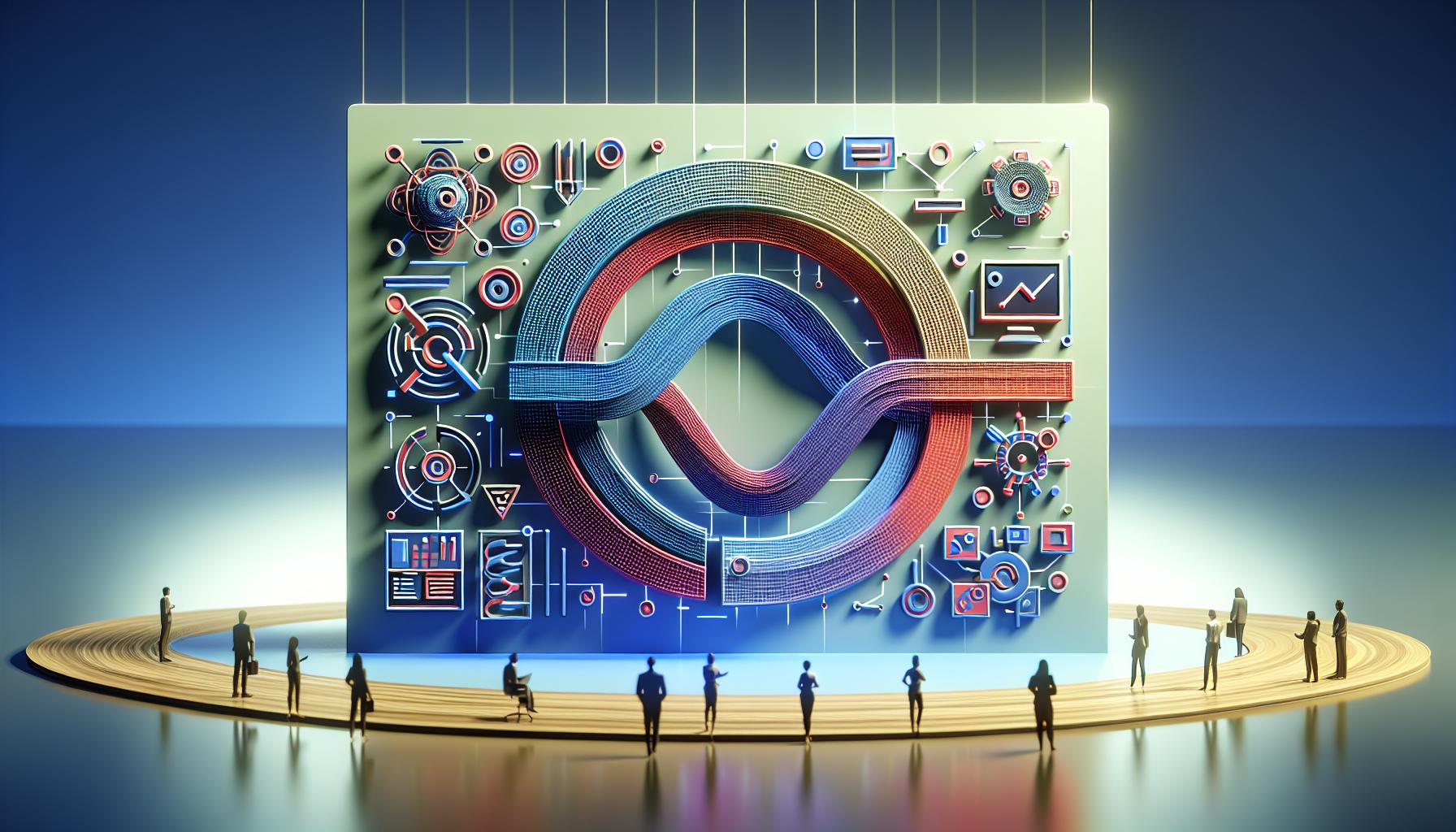
Introduction
The landscape of Artificial Intelligence (AI) is continually evolving, demanding constant refinement of A.I. models to stay at the cutting edge. This article delves into the iterative process of A.I. model improvement, crucial for achieving and maintaining peak performance. Using OpenAI technologies as a case study, we explore structured methodologies businesses can adopt to enhance their A.I. implementations effectively.
The Importance of Iteration in A.I. Development
In the realm of Artificial Intelligence, iteration refers to the cyclical process of refining A.I. models. This involves an ongoing loop of design, deploy, evaluate, and refine, ensuring that each model not only meets its initial goals but evolves with emerging requirements and data scenarios. Iteration is vital for adapting to complex, real-world environments and for continual learning where A.I. models improve with increased data and tweaked algorithms.
Setting Up Effective Evaluation Systems
Before embarking on the iterative process, it's crucial for businesses to set up robust evaluation systems. These systems assess the performance of A.I. models against a set of benchmarks or Key Performance Indicators (KPIs). Effective evaluation begins with defining clear, measurable goals that align with business objectives. Involving a mix of technical and domain experts can also enhance the objectivity and effectiveness of the evaluation process.
Choosing the Right Improvement Techniques
The selection of appropriate techniques for refining A.I. models is crucial. Techniques can range from data augmentation, which enhances the diversity and quantity of training data, to algorithm tuning and neural architecture search (NAS), which look for optimal model structures. Furthermore, reinforcement learning can be applied to some models to allow them to learn from each interaction. The choice of technique often depends on specific model requirements and organizational goals.
Iterative Refinement Based on Performance Data
Performance data gathered during evaluations informs the iterative improvement of A.I. models. This data-driven approach ensures objectivity in optimizing models. Businesses should focus on incorporating automated feedback loops that collect and analyze data continuously, allowing them to observe impacts almost in real-time. This ongoing analytic approach helps in making informed decisions about when and how to adapt A.I. models efficiently.
Real-World Case Studies of A.I. Improvement
Several leading organizations have successfully implemented iterative model enhancement using OpenAI technologies. These case studies often highlight substantial improvements in model accuracy, efficiency, and adaptability to new scenarios. For instance, an e-commerce company may use continual iteration to refine its recommendation systems, leading to higher conversion rates and customer satisfaction. Another example could be a healthcare provider using A.I. models to predict patient outcomes more accurately, adapting to new clinical data as it becomes available.
Conclusion
The journey towards A.I. perfection is unending. By embracing an iterative approach and leveraging the capabilities of advanced OpenAI technologies, businesses can enhance their A.I. models to better meet both present and future demands. This continual improvement not only boosts performance but also empowers innovations, setting a company apart in today's tech-driven marketplace.