Google Faces Challenges with AI Summaries: Accuracy Issues and Steps for Improvement
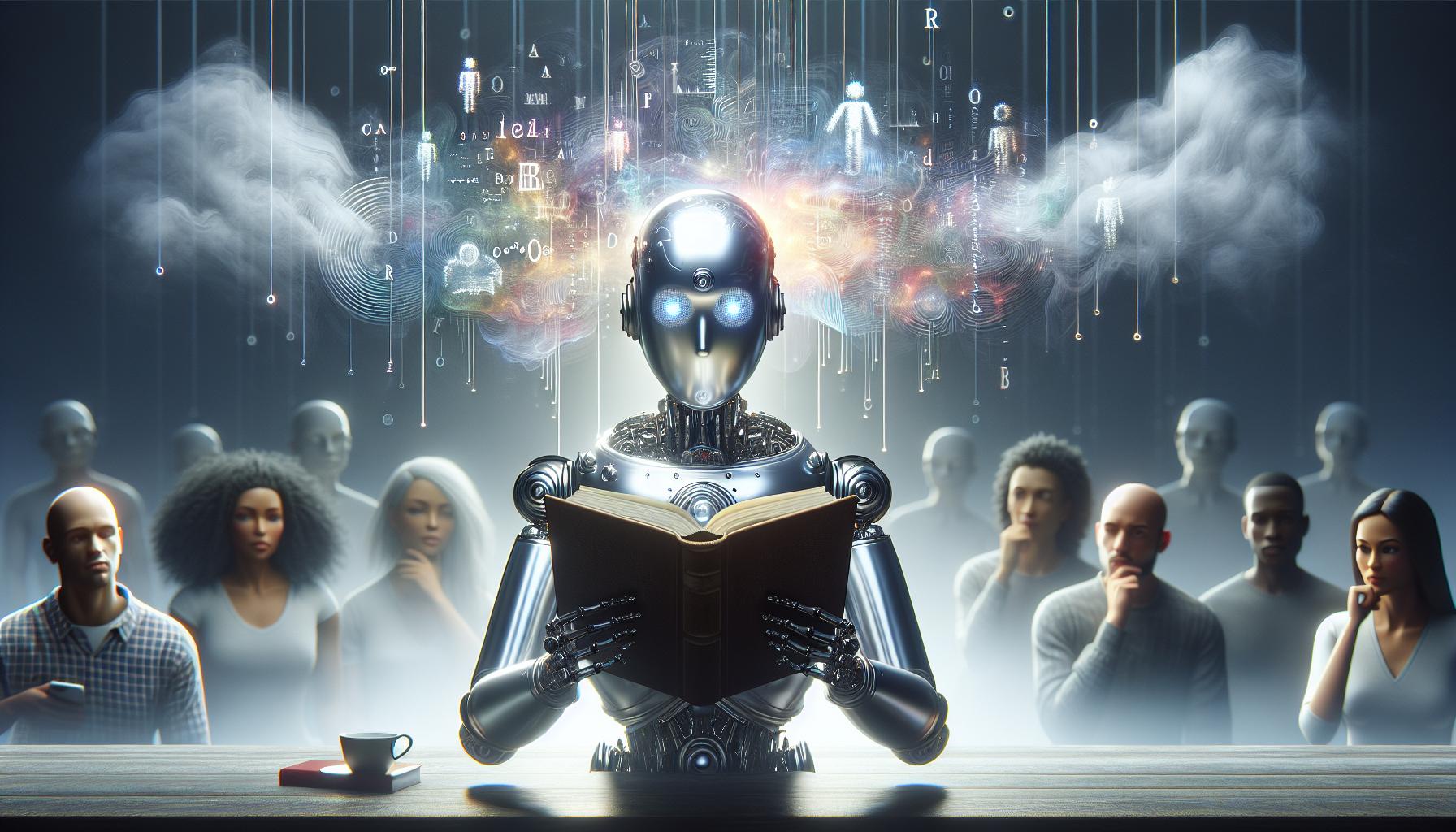
Introduction
Google's A.I. Overviews feature, designed to summarize vast amounts of information quickly and efficiently, is facing significant scrutiny. While the technology aims to streamline information consumption, the issue of accuracy has become a critical concern. Google's CEO Sundar Pichai acknowledges this problem, attributing it to a phenomenon known as hallucinations, which are common in A.I. large language models. Despite this, Pichai remains optimistic about the overall utility and progress of A.I. Overviews. This article explores the nature of these inaccuracies, Google's efforts to address them, and some real-world implications of these AI-generated errors.
The Problem of A.I. Hallucinations
AI hallucinations refer to instances where Artificial Intelligence systems generate information that is incorrect, misleading, or completely fabricated. This is particularly problematic when the A.I. is tasked with summarizing complex or nuanced information. Google's A.I. Overviews are no exception. The inaccuracies have led to widespread criticism and raised questions about the reliability of Google Search as a whole.
How Widespread Are the Inaccuracies?
Inaccuracies in AI-generated summaries are not isolated incidents but widespread. Users have reported numerous cases where the A.I. has provided incorrect information, sometimes with serious implications. These errors can range from minor factual inaccuracies to significant distortions of the original information. The extent of these inaccuracies has prompted Google to take the issue seriously, but as Sundar Pichai has noted, there is currently no foolproof solution to eliminate these hallucinations entirely.
Examples of Hallucinations
Sundar Pichai has provided several examples of hallucinations to illustrate the gravity of the problem. In one instance, the A.I. summarized a scientific article in a way that misrepresented the original research findings. In another case, the A.I. generated historical summaries that contained dates and events that never actually occurred. These examples highlight the potential dangers of relying on AI-generated summaries, particularly in areas where accuracy is paramount.
Steps Google Is Taking to Improve Accuracy
Despite the ongoing challenges, Google is actively working on several fronts to improve the accuracy of its A.I. Overviews:
1. Enhanced Data Training
Google is investing in more extensive and higher-quality datasets to train its A.I. models. By providing the A.I. with a broader and more reliable base of information, the company hopes to reduce the frequency of inaccuracies.
2. Human Oversight
Another measure involves incorporating more human oversight into the AI's decision-making process. Human reviewers are employed to cross-check AI-generated summaries for accuracy, particularly in high-stakes fields like medicine and law.
3. Algorithm Refinement
Google is continually refining its algorithms to better understand context and nuances. This involves improving the AI's natural language processing capabilities to minimize the risk of generating misleading or incorrect information.
4. User Feedback Mechanisms
Google has implemented mechanisms for users to report inaccuracies directly. This feedback is invaluable for identifying recurring issues and areas where the A.I. struggles most.
Challenges and Future Directions
While these steps are promising, the problem of A.I. hallucinations is far from resolved. The complexity of language and the subtleties of meaning pose ongoing challenges. However, the advancements in A.I. technology and Google's commitment to improving its systems offer hope for more reliable AI-generated summaries in the future.
Conclusion
Google's A.I. Overviews feature represents a significant advancement in the way we consume information, but it is not without its flaws. The issue of hallucinations and inaccuracies is a serious concern that Google is actively addressing through enhanced data training, human oversight, algorithm refinement, and user feedback mechanisms. While the road to perfect accuracy is long, the steps being taken now are crucial for building more reliable A.I. systems in the future.