Evaluating AI Hallucinations: Galileo's Hallucination Index for Gen AI LLMs
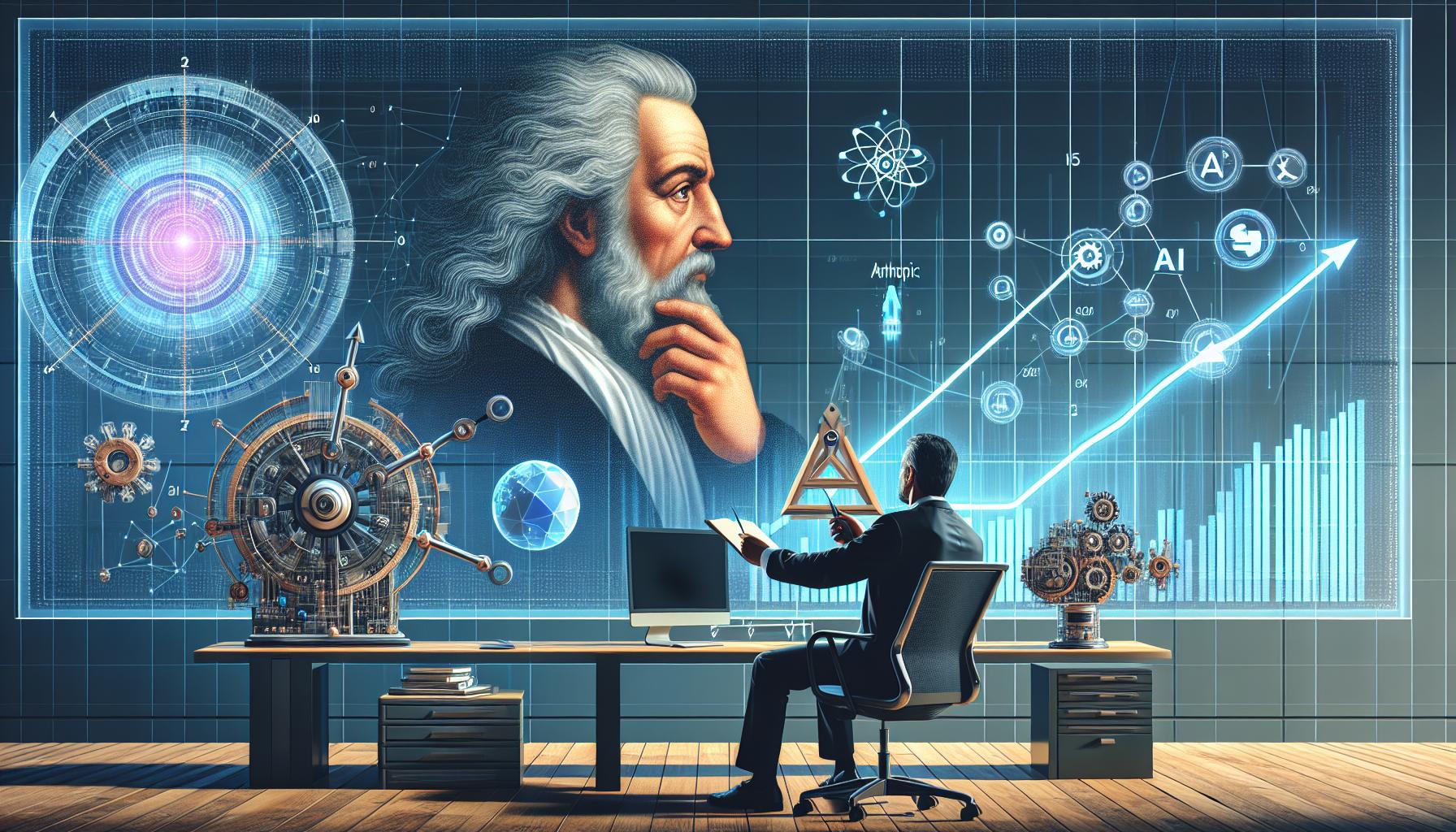
Introduction
In the rapidly evolving world of Artificial Intelligence, addressing AI hallucinations—or inaccuracies in AI-generated content—is a critical challenge for enterprises. Galileo, a leader in generative AI for enterprises, has recently unveiled its latest Hallucination Index. This Index evaluates 22 significant Generative AI Large Language Models (LLMs) from leading companies such as OpenAI, Anthropic, Google, and Meta. The goal is to provide a comprehensive understanding of how these models perform, particularly in terms of accuracy and cost-effectiveness.
What is Galileo's Hallucination Index?
Galileo's Hallucination Index serves as a benchmark for measuring the performance of various Generative AI LLMs. It applies Galileo's proprietary metric, known as context adherence, to evaluate how accurately these models produce outputs across a range of input lengths—from 1,000 to 100,000 tokens. This metric aims to help enterprises make informed decisions by balancing costs with performance.
Who's Winning Against AI Hallucinations?
This year, the Hallucination Index doubled its model count, adding 11 new models, thereby reflecting the rapid expansion of both open- and closed-source LLMs within the past eight months. Among the top performers were:
- Anthropic's Claude 3.5 Sonnet: This model emerged as the top overall performer, demonstrating near-perfect scores across varying context lengths.
- Google's Gemini 1.5 Flash: Recognized as the most cost-effective model, excelling across various tasks.
- Alibaba's Qwen2-72B-Instruct: Highlighted as the best open-source model, particularly for short and medium context scenarios.
Trends in the LLM Landscape
Several key trends have emerged from this year's Hallucination Index:
- Open-source models: These models are becoming increasingly competitive, offering improved performance at reduced costs.
- RAG LLMs: Retrieval Augmented Generation LLMs show marked improvement in managing extended contexts without compromising quality.
- Smaller models: Sometimes, smaller models can surpass larger ones in performance efficiency.
- Global competition: The global landscape is intensifying, with emerging strong players like Mistral's Mistral-large and Alibaba's Qwen2-72B-Instruct.
Despite the dominance of closed-source models, the evolving landscape suggests robust competition, especially with strong performers from outside the US. For example, while Google's open-source Gemma-7b model struggled, its closed-source Gemini 1.5 Flash consistently ranked high.
Understanding Context Adherence
One of the unique features of Galileo's Hallucination Index is its use of the context adherence metric. This proprietary metric measures the accuracy of AI outputs by assessing how well the AI systems generate content in relation to the given context. Context adherence is vital for enterprises that rely on AI for generating precise and relevant outputs, thereby reducing the risk of AI hallucinations.
Superior Performance of Closed-Source Models
Closed-source models like Anthropic's Claude 3.5 Sonnet have shown superior performance due to several factors:
- Resource Allocation: Closed-source models often have more resources for research and development, leading to better optimization.
- Data Quality: Access to high-quality, proprietary datasets allows these models to train more effectively.
- Advanced Algorithms: The use of advanced algorithms and architectures that are not publicly disclosed can lead to better performance.
Closing the Gap: Open-Source Models
Open-source models are closing the performance gap with their closed-source counterparts through various means:
- Community Collaboration: Open-source models benefit from a community of developers and researchers who contribute to their improvement.
- Innovation in Training Techniques: New and innovative training techniques are being applied to open-source models, enhancing their performance.
- Cost-Effectiveness: The reduced cost of implementation makes open-source models an attractive option for many enterprises.
Balancing Performance and Budget
For enterprises, the key to effective AI implementation lies in balancing performance with budgetary considerations. Galileo's Hallucination Index serves as an essential tool in this regard, offering insights into which models provide the best return on investment. By understanding the nuances of each model's performance, enterprises can make more informed decisions that align with their specific needs and financial constraints.
Upcoming Industry Events
For those looking to gain deeper insights into the evolving landscape of AI, several industry events are on the horizon. Events like the AI & Big Data Expo in Amsterdam, California, and London provide opportunities to explore advancements in intelligent automation, digital transformation, and cybersecurity. These comprehensive events are co-located with other leading conferences, offering a unique platform for networking and knowledge-sharing among industry leaders.
The Galileo hallucination index typically uses several key indicators to assess the likelihood of hallucinations in AI-generated content. These indicators include:
Factual Accuracy: Measures whether the generated content aligns with verifiable real-world facts. This is usually checked against databases, knowledge sources, or authoritative references.
Consistency: Evaluates whether the AI’s output is internally consistent and coherent across different parts of a response or related queries. Inconsistent outputs often indicate hallucinations.
Reference Anchoring: Assesses whether the AI appropriately references data points or sources when generating factual content. A lack of referencing or incorrect references can indicate hallucination.
Logical Soundness: Examines whether the conclusions or reasoning in the generated content follow a logical pattern. Illogical reasoning may indicate that the AI is making up information.
Contextual Relevance: Checks whether the AI remains on-topic and appropriately interprets the input context. When the model strays from the context, it is often a sign of hallucination.
Cross-Validation with External Data: In some implementations, outputs are cross-validated with external databases or APIs to determine if the generated content matches real-world information.
Language Patterns: Tracks certain linguistic or stylistic patterns that have been identified as common in hallucinated outputs, such as overconfidence in statements that are unsupported or vague phrasing.
By using these indicators, the Galileo hallucination index can systematically evaluate how often and under what conditions the AI produces outputs that are detached from reality.
Conclusion
As AI continues to evolve, the issue of hallucinations remains a significant challenge. However, tools like Galileo's Hallucination Index are helping enterprises navigate this complex landscape. By evaluating the performance of various Gen AI LLMs, this Index provides valuable insights that aid in making informed decisions, balancing cost with performance, and ultimately enhancing the reliability of AI implementations.