Anthropic's Funding Program for AI Benchmark Development: Criteria, Differences, and Expected Impacts
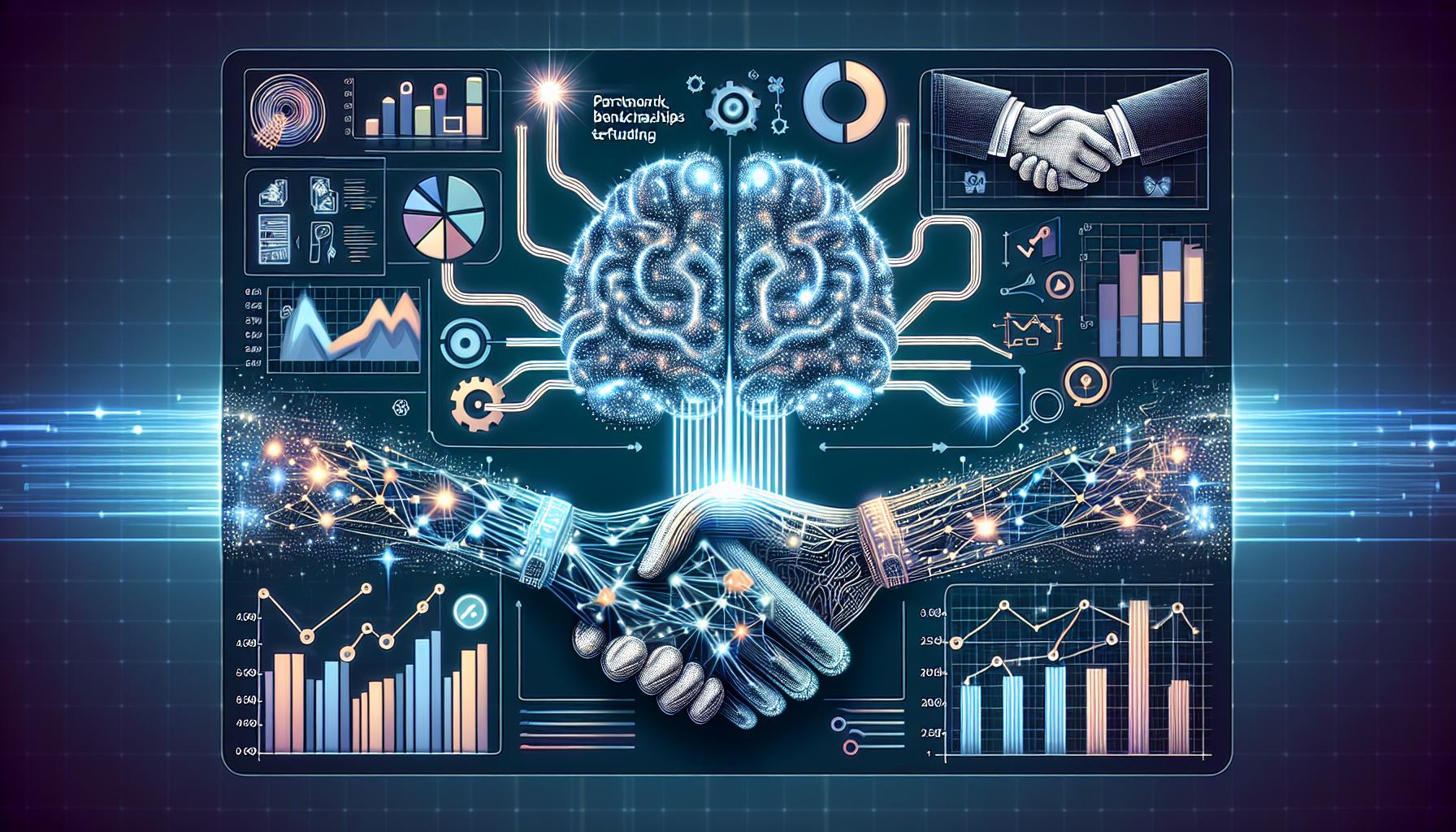
Introduction
In an exciting development within the Artificial Intelligence (AI) community, Anthropic has announced a new funding program to foster the creation of benchmarks that can evaluate A.I. models effectively. This initiative, highlighted in a recent company blog post, focuses mainly on generative models such as Claude, one of Anthropic's A.I. systems. The primary goal of this program is to support third-party organizations in developing benchmarks that can assess both the performance and the broader impacts of these A.I. systems. This article explores the criteria for funding, how these new benchmarks will differ from existing methods, and the anticipated impacts on A.I. model development and deployment.
Criteria for Receiving Funding
The success of Anthropic's funding program hinges on the careful selection of third-party organizations capable of developing effective A.I. benchmarks. While Anthropic has not disclosed the complete list of criteria for funding, we can speculate based on common industry practices and the stated goals of this initiative. Firstly, organizations applying for funding must demonstrate a robust understanding of A.I. systems, particularly generative models. This means having a team with deep expertise in machine learning, A.I. ethics, and software development.
Secondly, the proposed benchmarks must be innovative and capable of addressing current gaps in A.I. evaluation. This involves showing how their benchmarks can measure aspects of A.I. performance and impact that existing methods might overlook. Thirdly, applicants will likely need to provide a feasible plan for developing these benchmarks, including clear timelines, resource allocation, and methods for testing and validation. Lastly, given the focus on broader impacts, organizations must demonstrate how their benchmarks can contribute to a more comprehensive understanding of how A.I. systems affect various socio-economic factors.
Differences from Existing A.I. Evaluation Methods
One of the main motivations behind Anthropic's funding program is to push the boundaries of current A.I. evaluation methods. Traditional benchmarks often focus on specific metrics such as accuracy, speed, and computational efficiency. While these are important, they offer a limited view of an A.I. model's overall performance and impact. The new benchmarks funded by Anthropic aim to go beyond these narrow metrics.
First and foremost, these benchmarks will be more holistic, considering a wider range of factors that influence A.I. performance. For example, they may include social impact assessments, which examine how A.I. systems affect human behavior, equity, and ethical considerations. They might also look at long-term performance metrics, observing how A.I. models evolve and adapt over time under different conditions. Additionally, transparency and explainability will likely be key components of these new benchmarks. Traditional evaluation methods often treat A.I. models as black boxes, focusing solely on input and output without considering the interpretability of the underlying processes. In contrast, the new benchmarks will emphasize understanding the decision-making processes of A.I. models, making it easier to identify biases, errors, and areas for improvement.
Expected Impacts on A.I. Development and Deployment
The introduction of new, more comprehensive benchmarks is expected to have a significant impact on the development and deployment of A.I. models. One immediate effect will likely be a shift in research priorities within the A.I. community. As new benchmarks highlight previously overlooked aspects of A.I. performance and impact, researchers and developers will adapt their focus to meet these new standards. This could lead to innovations in A.I. model architecture, training methodologies, and evaluation techniques.
Moreover, the emphasis on broader impacts will foster a more socially responsible approach to A.I. development. Organizations will be incentivized to consider the ethical implications of their A.I. systems, leading to models that are not only more effective but also more equitable and transparent. This aligns with a growing trend in the A.I. community to prioritize fairness, accountability, and transparency (FAT) in A.I. development.
Another important impact is the potential for better regulatory compliance. As governments and international bodies continue to develop regulations for AI, having comprehensive and reliable benchmarks will help organizations demonstrate compliance with these regulations. This can facilitate smoother deployment of A.I. systems across different sectors, from healthcare and finance to education and entertainment.
Finally, these new benchmarks will likely foster greater public trust in A.I. technologies. By providing more transparent and comprehensive evaluations of A.I. performance and impact, stakeholders—including consumers, policymakers, and business leaders—will have a clearer understanding of the benefits and risks associated with A.I. systems. This increased transparency can lead to more informed decision-making and a more nuanced public discourse around A.I. technologies.
Conclusion
Anthropic's funding program for A.I. benchmark development represents a significant step forward in the evaluation of generative A.I. models. By setting clear criteria for funding, focusing on innovative and comprehensive benchmarks, and anticipating broad impacts on A.I. development and deployment, Anthropic is paving the way for a more holistic understanding of A.I. performance and impact. As these new benchmarks are developed and implemented, we can expect to see a more responsible, equitable, and transparent A.I. landscape benefiting various aspects of society.